summer school:
16 -19 Sept 2024
Deep Learning for Language Analysis
Deadline: 16 June 2024
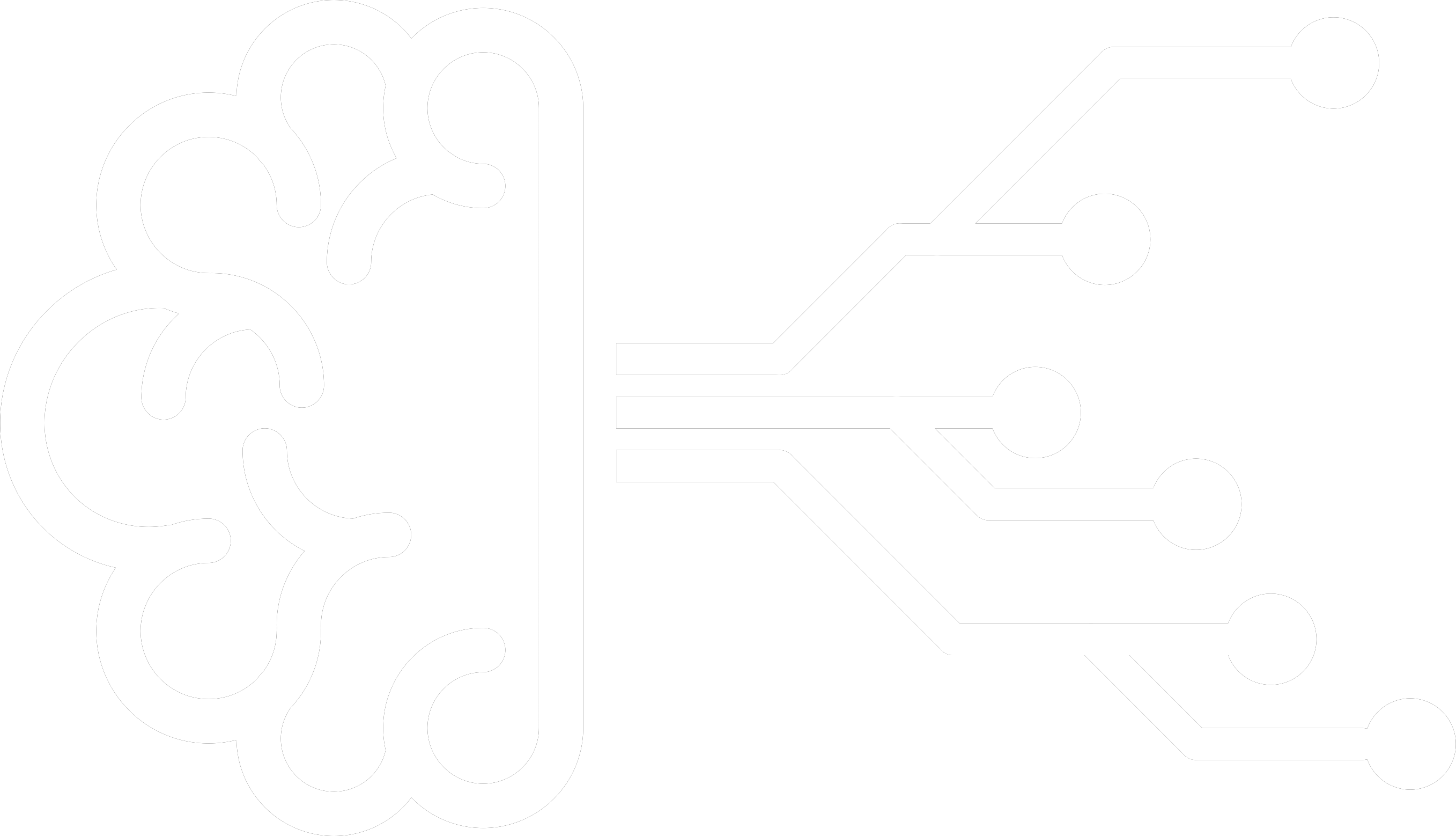
The summer school „Deep Learning for Language Analysis” addresses students and doctoral candidates from Linguistics and Digital Humanities, as well as other fields that are involved with the application of machine learning techniques. This year the summer school will be in presence again, taking place at the University of Cologne.
Participants can choose between two alternative workshop tracks:
- “Deep Learning with Audio & Speech Data”, given by Paul Wallbott
- “Prompting, Evaluation, Interpretation. An Introduction to LLMs in Text Analysis”, given by Axel Pichler (University of Stuttgart) and Janis Pagel (University of Cologne)
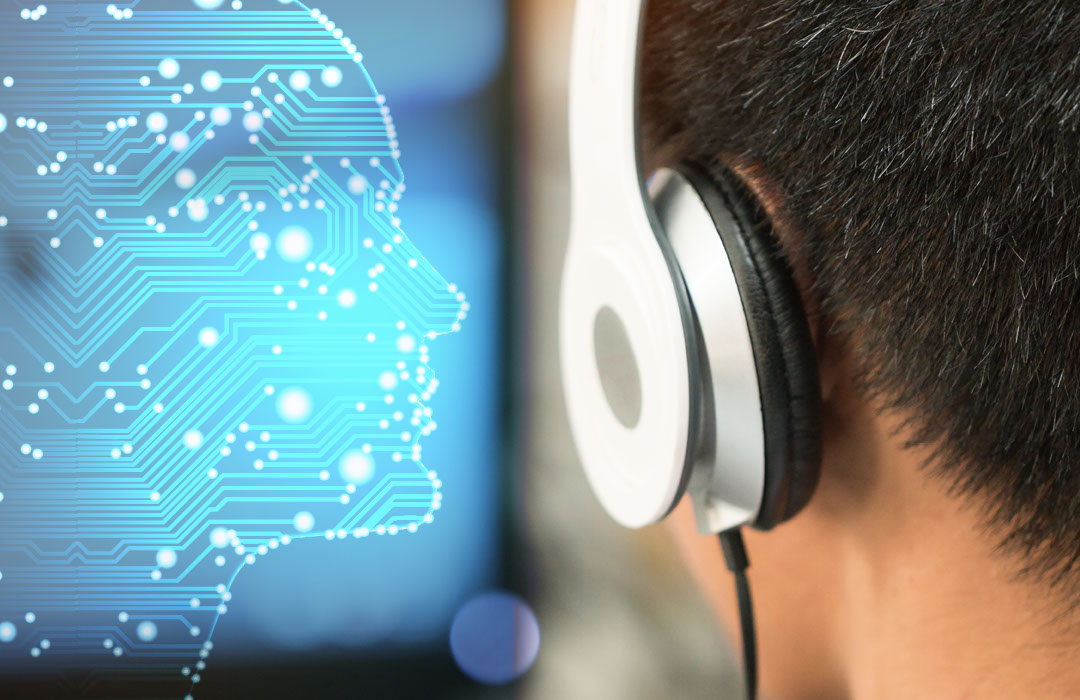
REGISTRATION
The school is mainly aimed at students and doctoral candidates from linguistics and digital humanities, but participants from other fields who are interested in machine learning are welcome to apply as well. Basic knowledge of Python is presumed.
To apply, please send an email to ml-school@uni-koeln.de by June 16th, 2024 with the subject „Registration – <Your Complete Name>“ and the following information (included in the email text)
a) Your highest academic degree including discipline or area (e.g. „BSc/MSc/PhD Linguistics“)
b) Information on your current academic position including discipline or area of work (e.g. „MSc/PhD/Postdoc linguistics/digital humanities“)
c) Your current Institution (e.g. „University of Cologne“)
d) A (very short) description of a project where you used Python. Previous knowledge of machine learning, if applicable.
e) Which one of the parallel tracks you want to attend (Audio or Text), or your preference if both are appealing to you.
f) 2-3 sentences explaining your motivation to attend the workshop.
There is no registration fee. The whole summer school will take place at the University of Cologne.
Deadline for registration: June 16th, 2024
Notification: July
Summer School: September 16th – September 19th 2024
Summer school Schedule
16-19 September 2024
Starting MON 16.09. at noon
Ending THU 19.09. at 3pm
A detailed schedule will follow soon!
Contact
The school is mainly aimed at students and doctoral candidates from linguistics and digital humanities, but participants from other fields who are interested in machine learning are welcome as well. Basic knowledge of Python is presumed.
Contact:
Organization
The school is funded by the University of Cologne’s Competence Area III
(CA3: Quantitative Modeling of Complex Systems) and organized in cooperation with the Center for Data and Simulation Science (CDS), the Department for Digital Humanities (IDH), the Cologne Center for eHumanities (CCeH), and the Data Center for the Humanities (DCH).
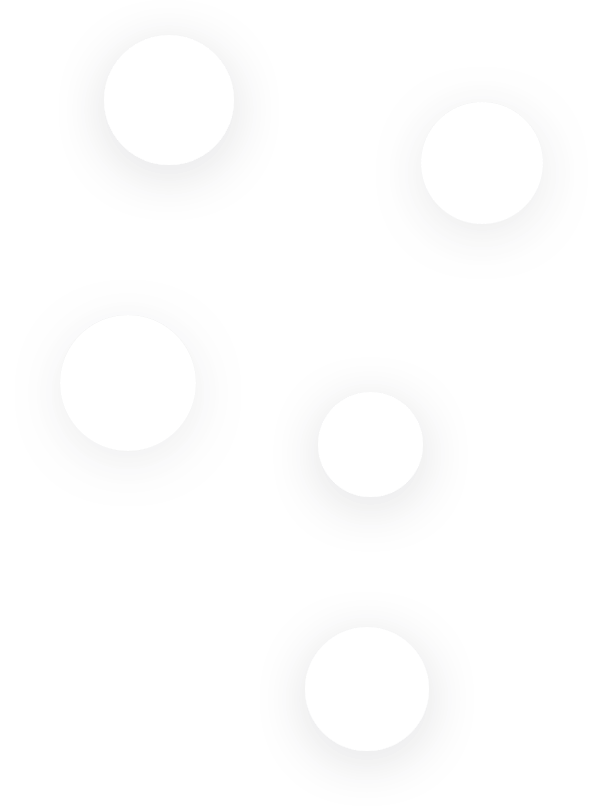
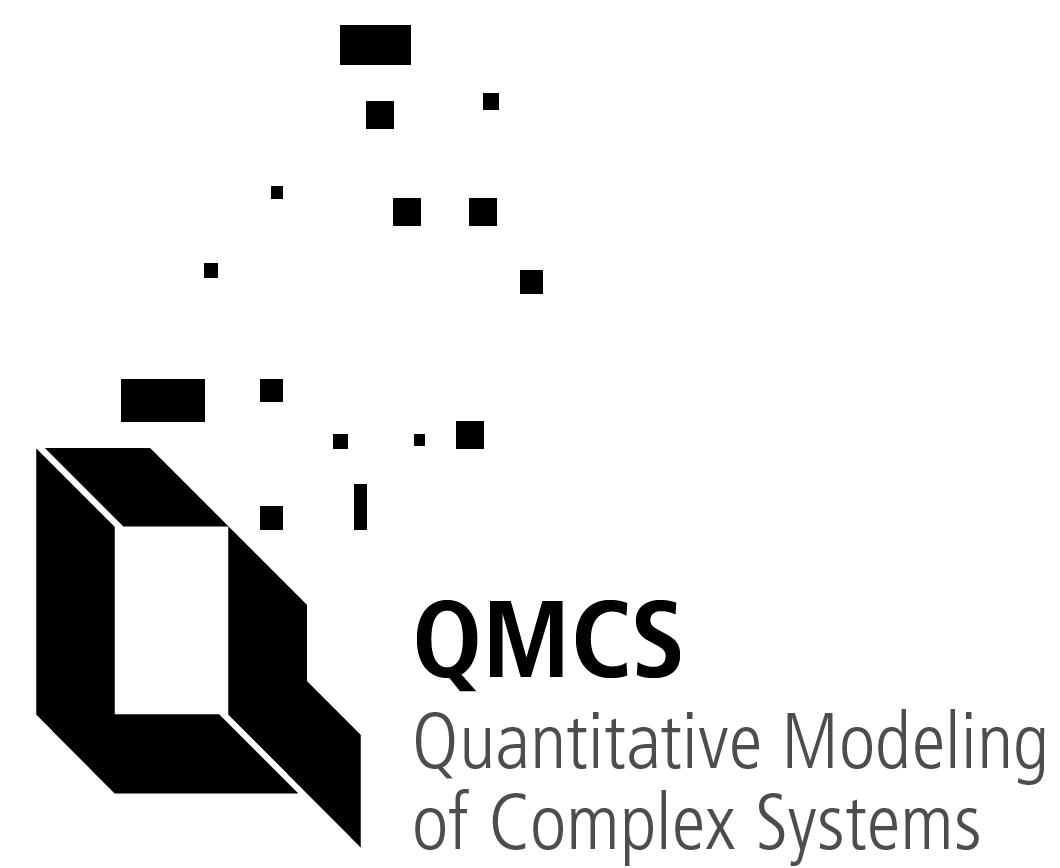
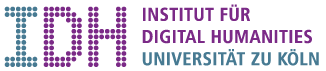
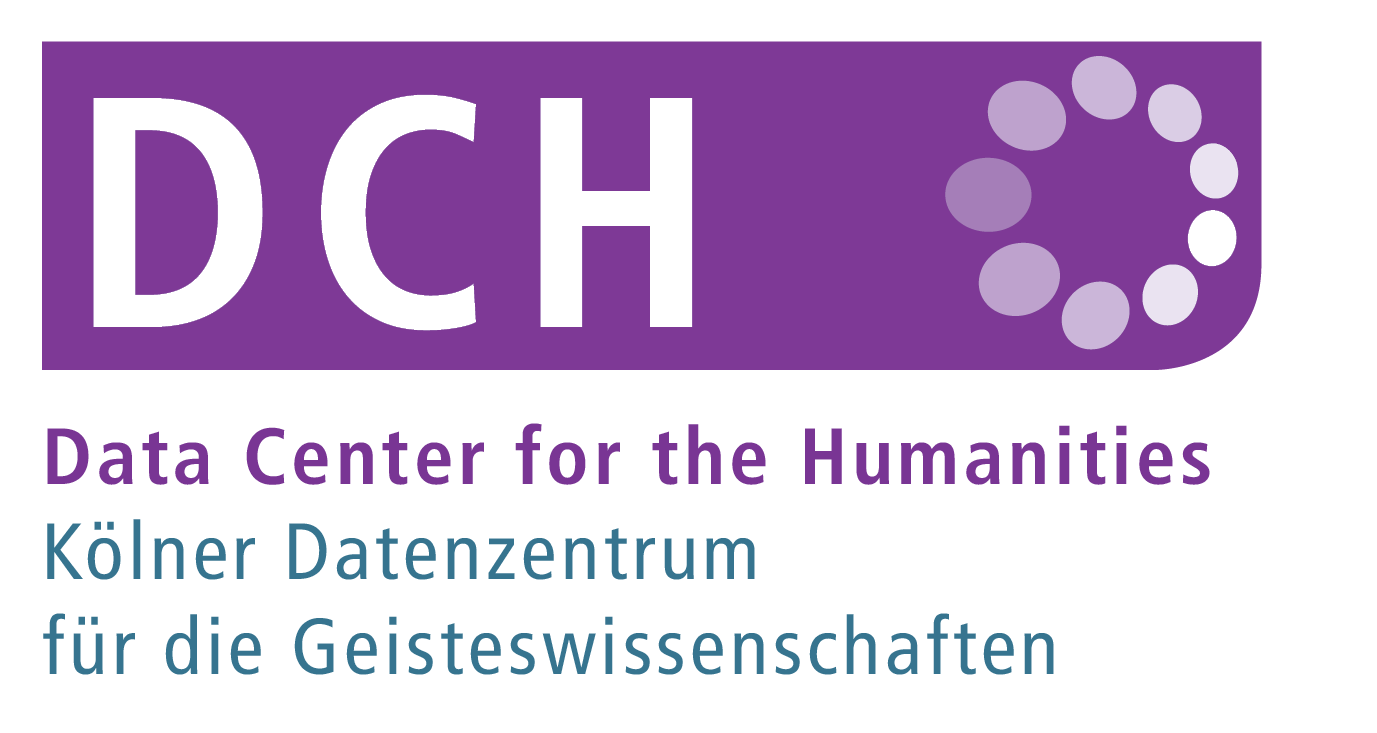
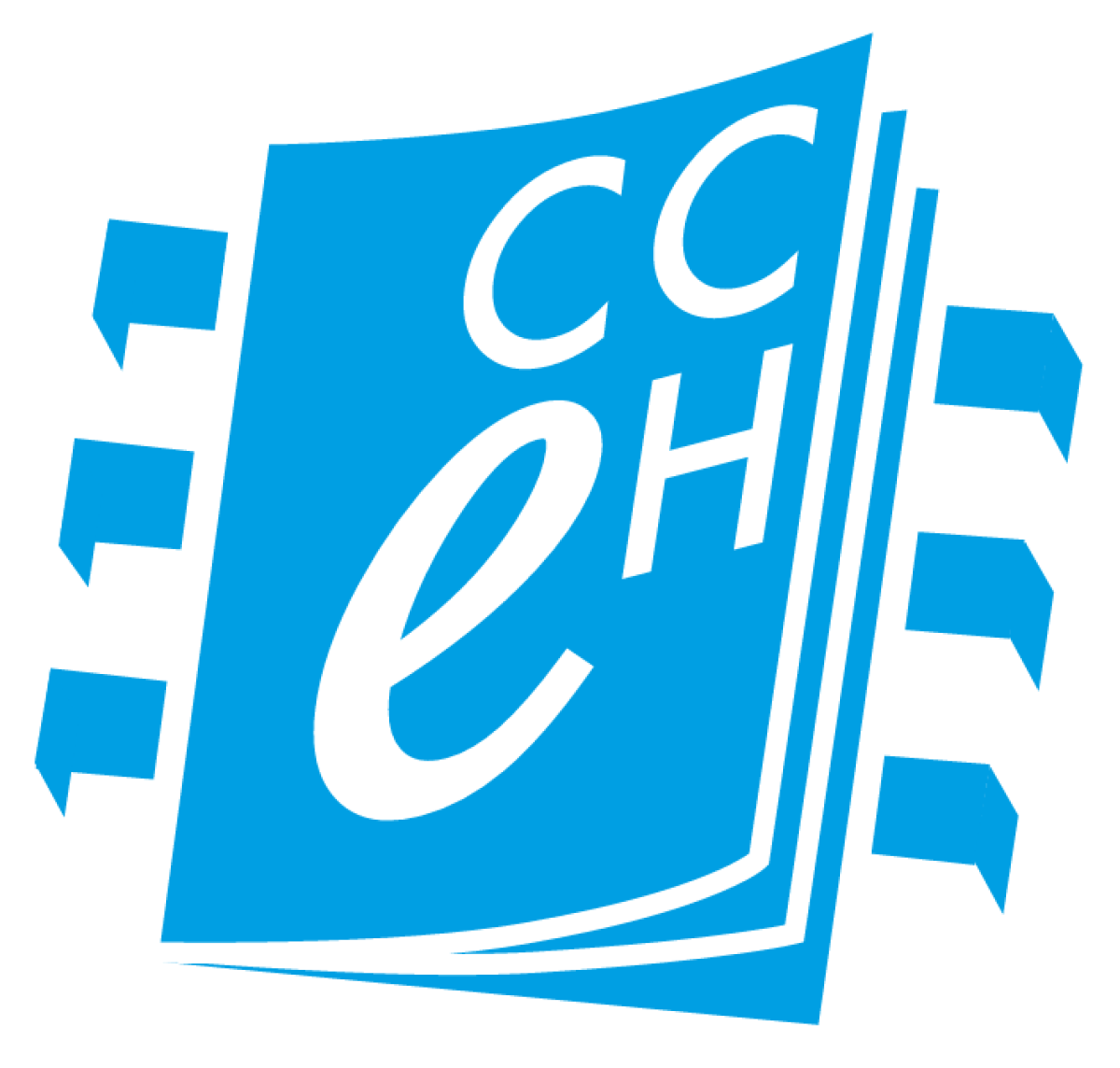
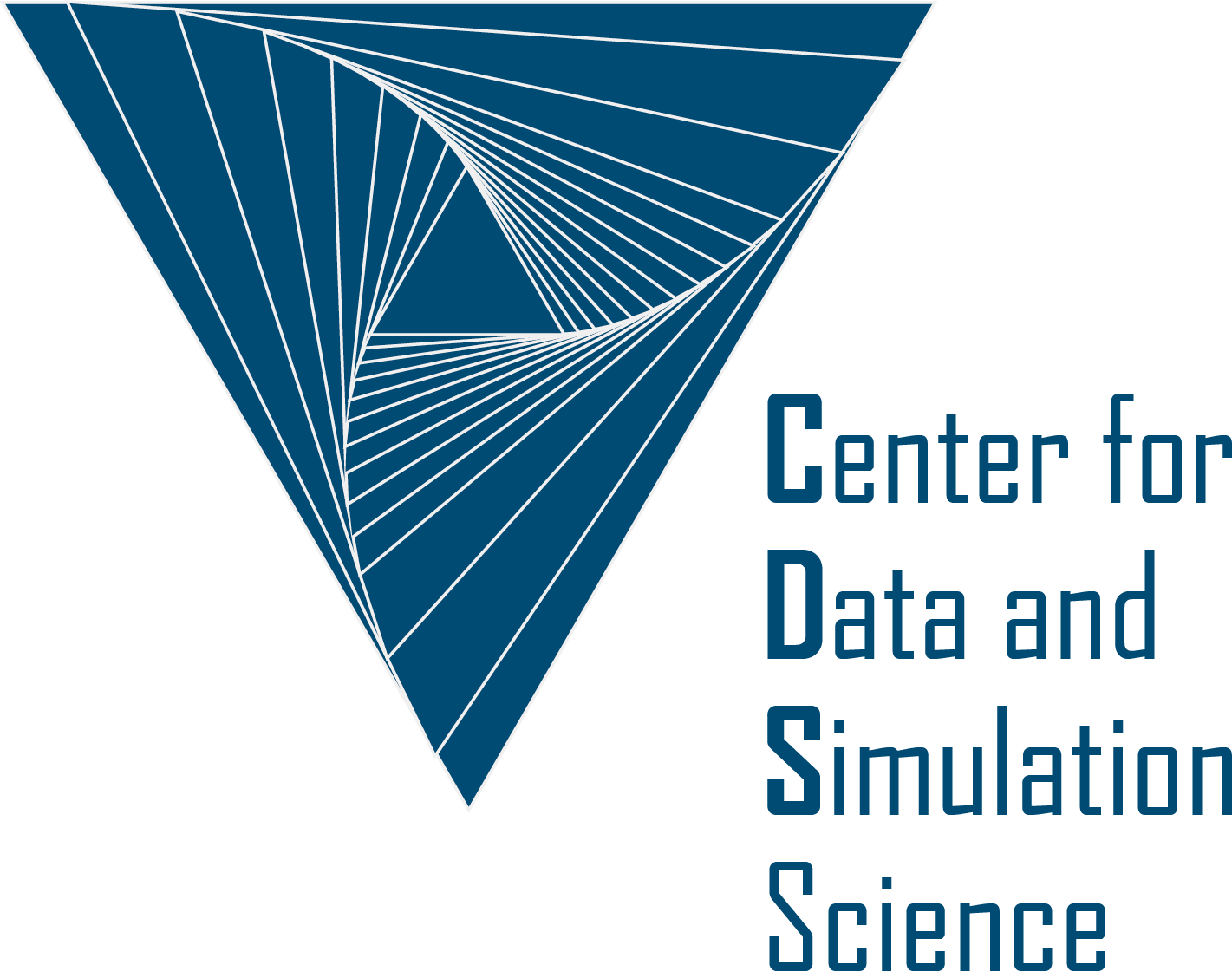